Kochava for AdvertisersAd Fraud Prevention
Defend Your Spend With AI-Powered Ad Fraud Prevention
Fight back against mobile & CTV ad fraud with the industry’s most trusted fraud detection tools, which analyze trillions of data points across millions of campaigns every day.
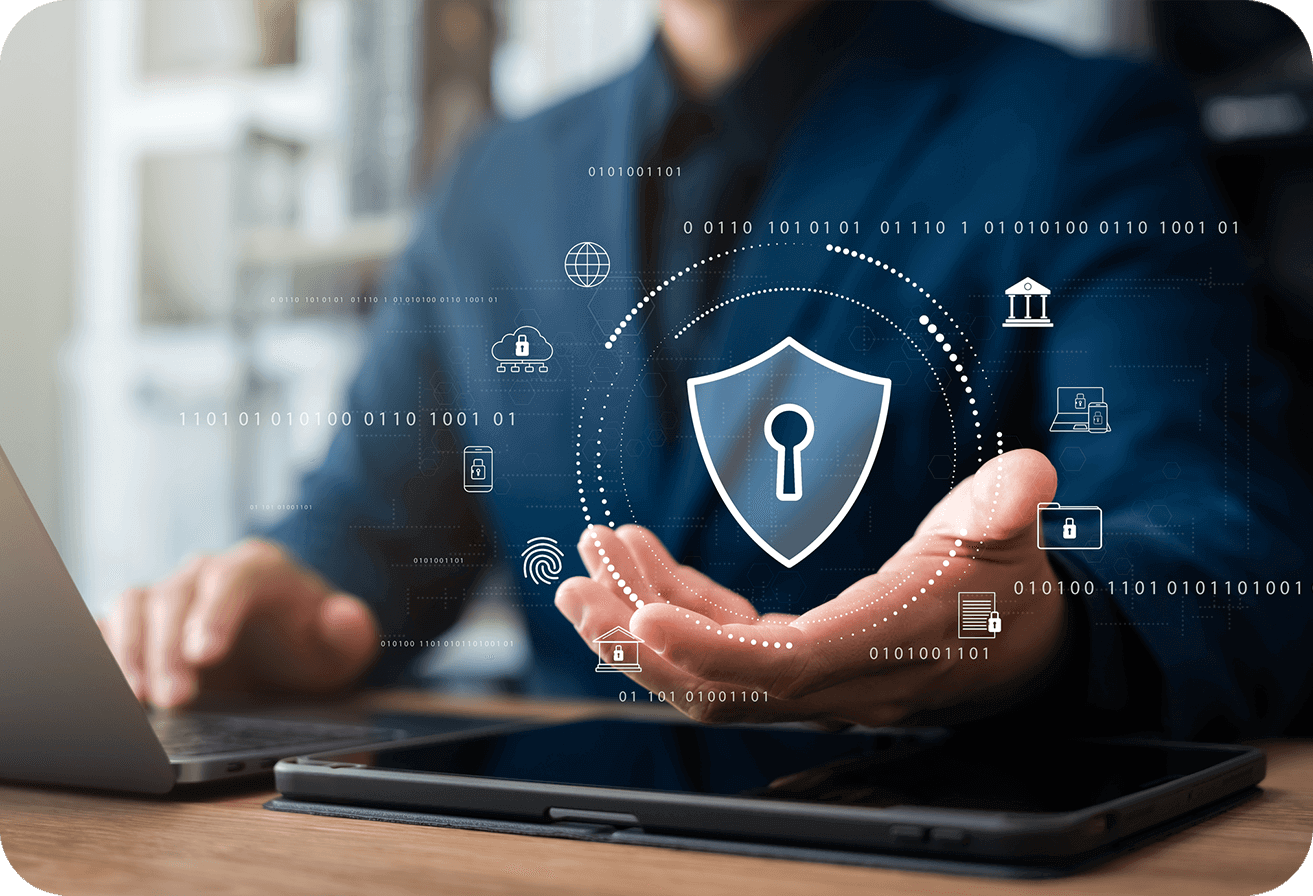
According to Juniper Research, ad fraud’s yearly price tag will hit $172 billion by 2028.
Refuse to become part of the statistic!
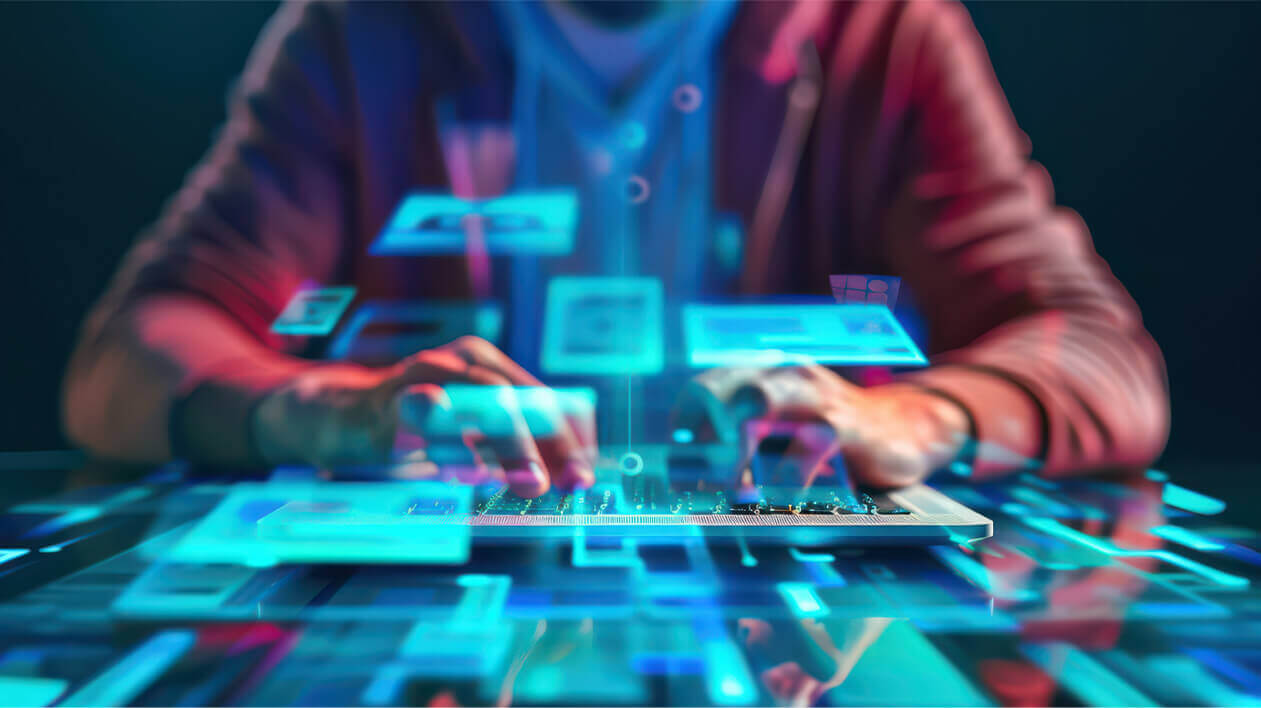
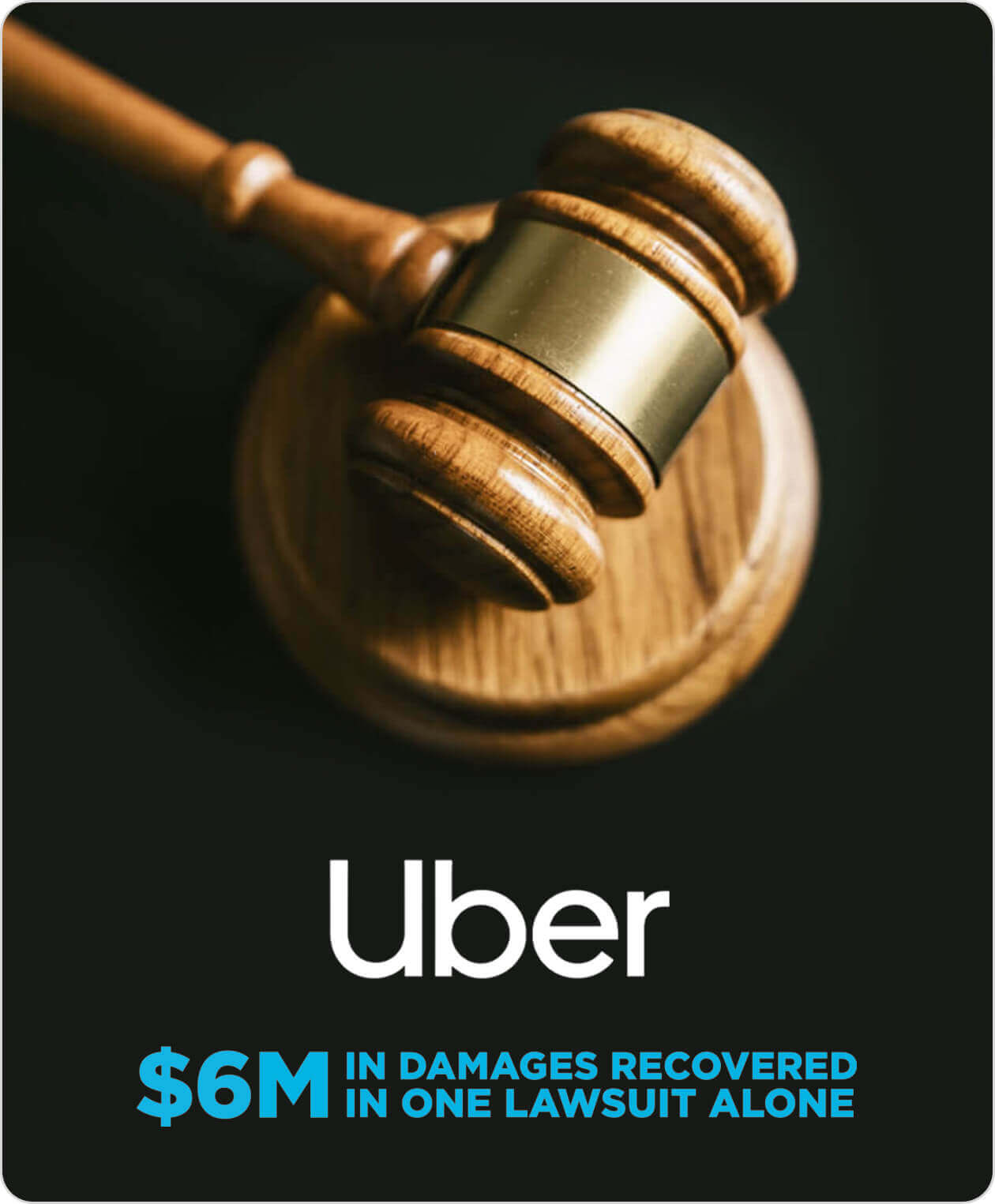
Aided by Kochava Experts, Uber Recovers Millions from Ad Fraud Scheme
“Kochava’s investigative fraud work was comprehensive and thorough. Their ability to illustrate the complex inner workings of digital ad fraud to the courtroom audience had a profound impact on the success of our litigation. Justice was served.”
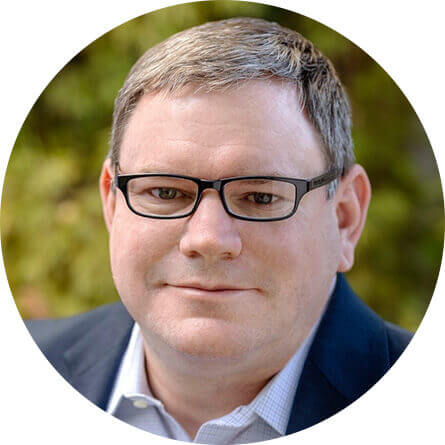
Ad Fraud Tactics We Prevent
Our multilayered, AI-powered tools detect and prevent all prevalent and emerging fraud tactics:
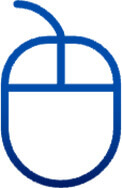
Click Injection
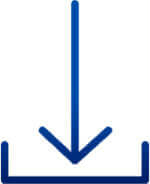
Install Hijacking
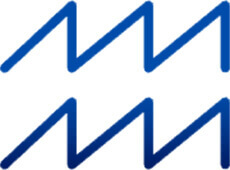
Click Flooding
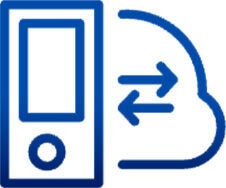
SDK Spoofing
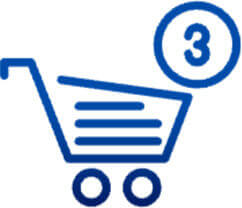
In-App Purchase Fraud
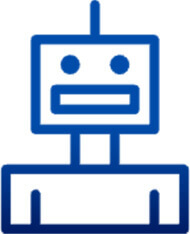
Fraud Bots
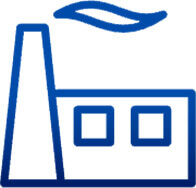
Device Farms
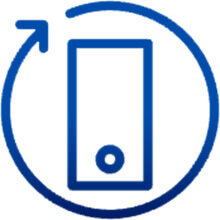
Device ID Rest
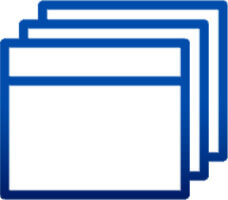
Ad Stacking
Diversify Your Defense Strategy
Get instant visibility into your compromised traffic with real-time fraud detection.
Activate the Kochava Global Fraud Blocklist at the flip of a switch to protect your campaigns seamlessly across all apps. Traffic that violates the blocklist is flagged for instant visibility in reporting and analytics, while also being disqualified from attribution to valid conversions.
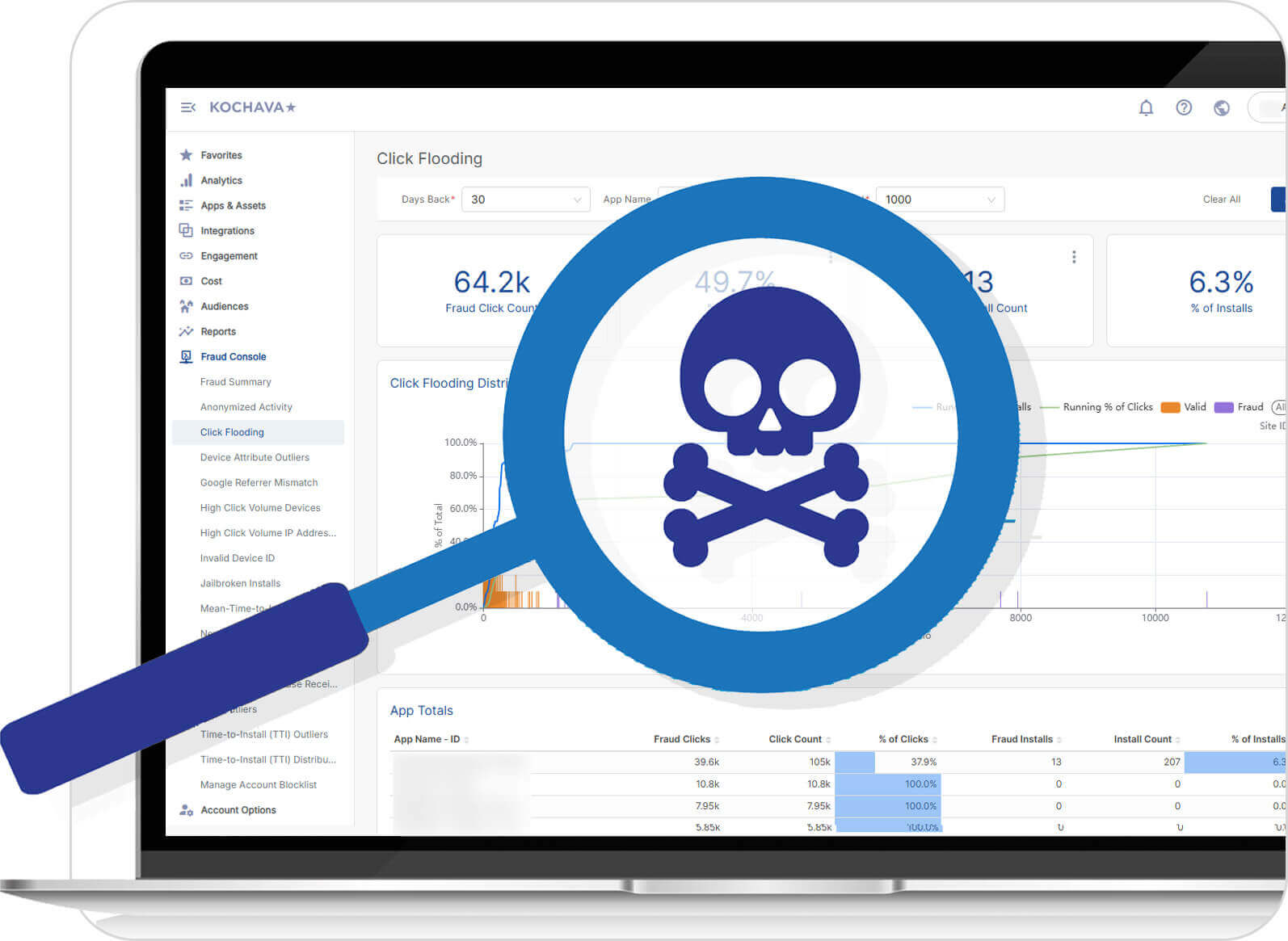
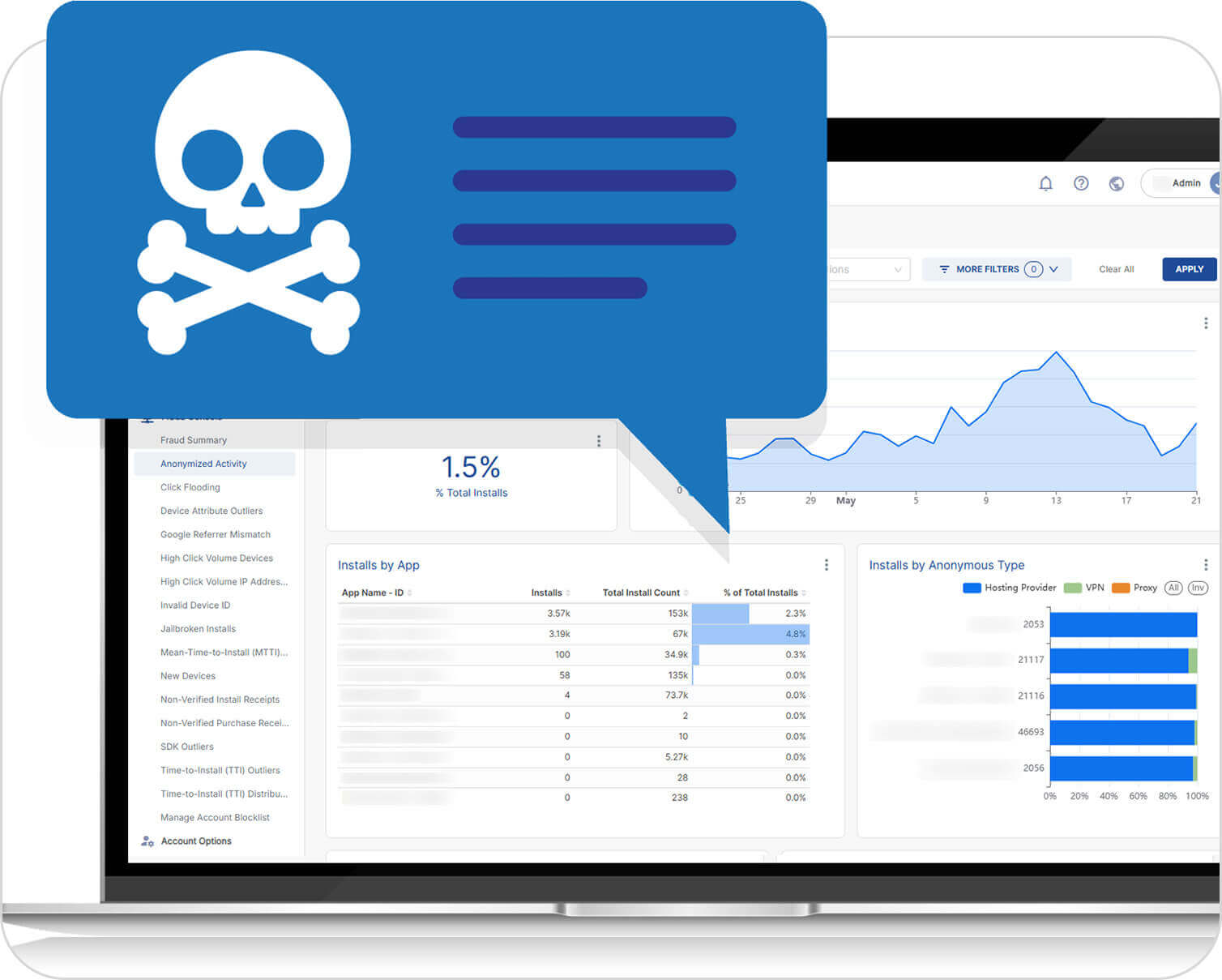
Customize thresholds and blocklists with personalized fraud alerts.
Go beyond one-size-fits-all solutions with customized fraud thresholding tailored to your brand’s needs. Personalize thresholding and account-level blocklisting to decide rules and dynamic conditions for blocking fraudulent sources. When these rules are violated, stay informed with immediate fraud alerting to know which media sources are flagged and blocked.
Set advanced rules to guard over your campaign traffic.
Kochava Traffic Verifier® enables you to configure traffic rules to enforce the terms of insertion orders (IOs) with media partners. Set rules for validation based on:
- Device characteristics
- Geography
- Campaign run dates
- Frequency capping
- iOS install receipts & Google UTM data
- and other criteria
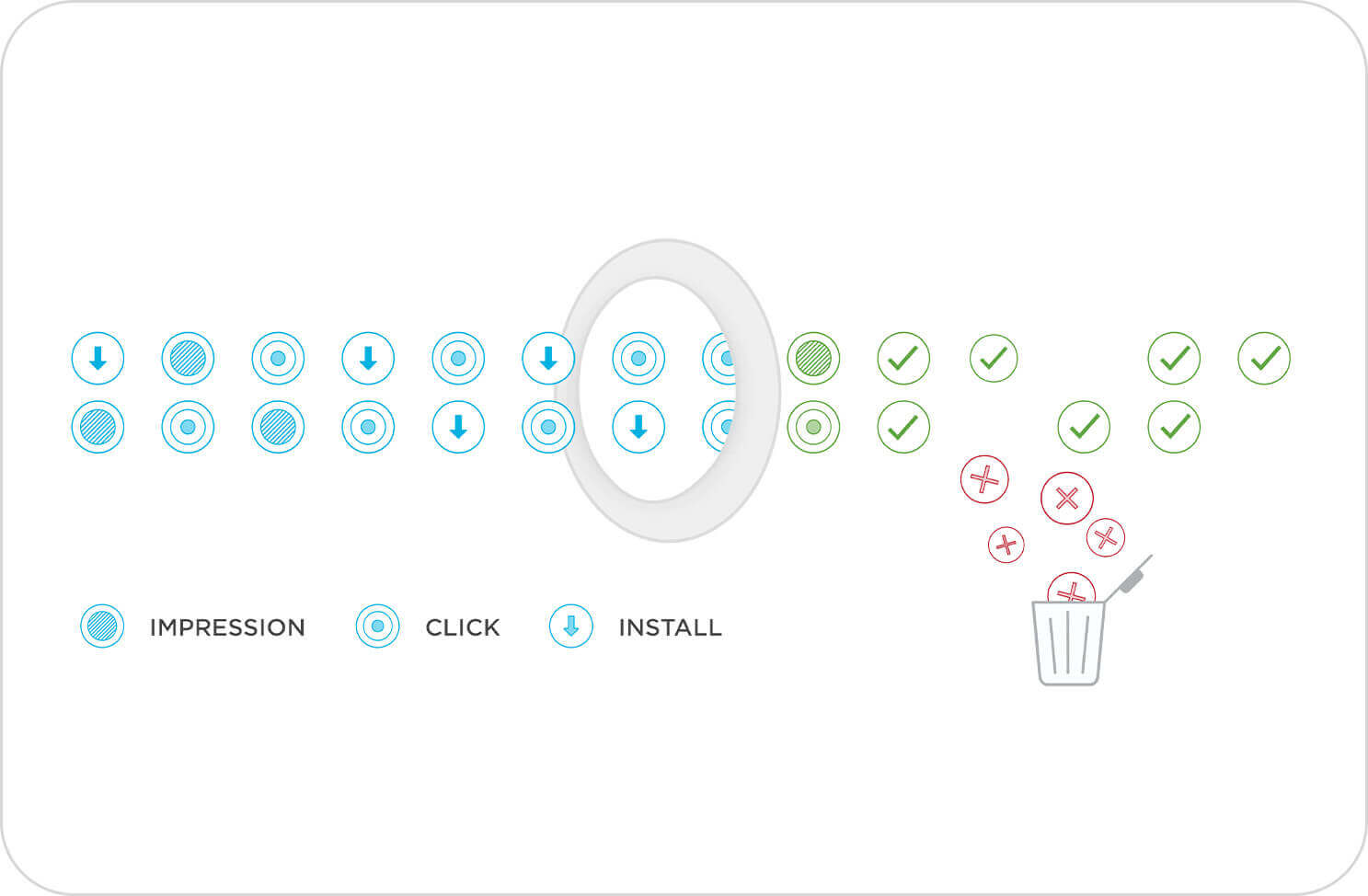
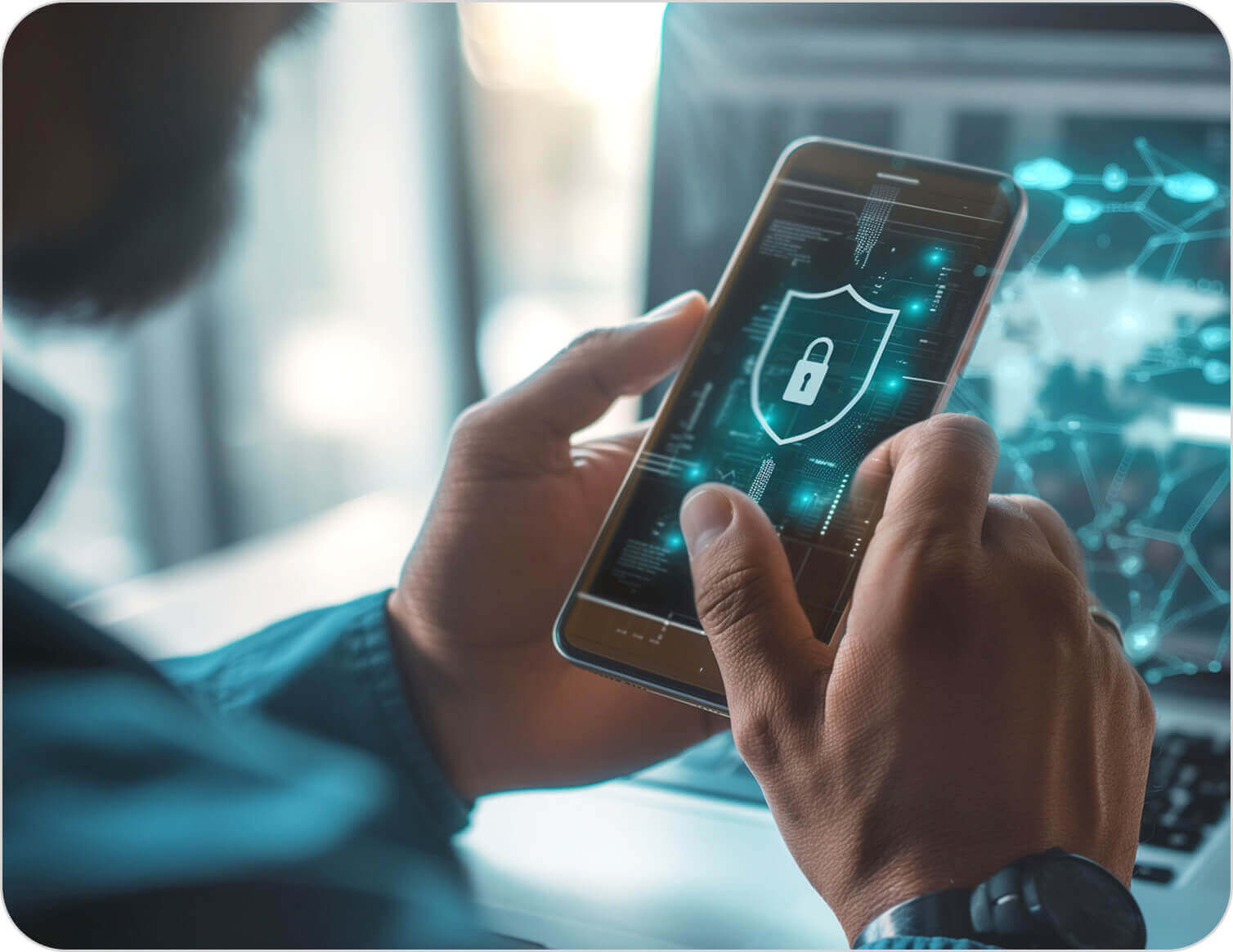
Stop SDK spoofing.
Detect and block spoofed data payloads to maintain the integrity of user engagement data and prevent cost-per-install & cost-per-event payouts on entirely fake conversions.
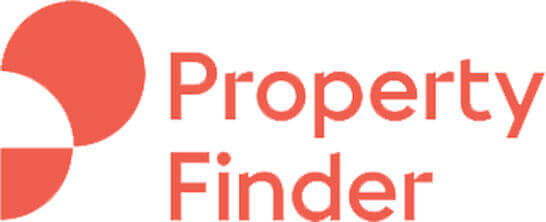
Property Finder Protects Their Ad Campaigns With Kochava
Over 90 days, Kochava Fraud Prevention blocked
2.15M fraudulent clicks
400K+ fake install payloads
“Kochava has provided us with a stable foundation of reliable measurement and fraud prevention from which we can truly scale our mobile app growth to better serve our consumers.”
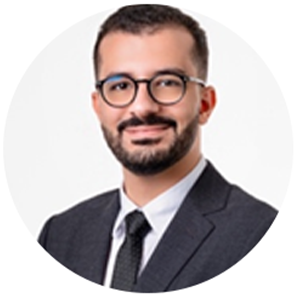