Enhancements to MMM make it a powerful tool for advertisers as privacy regulations evolve
As data and user privacy concerns continue to mount, advertisers are facing unprecedented challenges in collecting, analyzing, and utilizing customer data for targeted advertising. With consumers becoming more aware of their privacy rights, regulations like the EU’s General Data Protection Regulation (GDPR), California Consumer Privacy Act (CCPA), and Apple’s AppTrackingTransparency (ATT) framework have put strict limitations on data collection and usage.
It’s against this backdrop that marketing mix modeling (MMM), a practice that goes back more than half a century, is resurging as a powerful methodology to help marketers optimize their advertising strategies without overreliance on the one-to-one, device-level attribution data of last-touch attribution, the model that has dominated programmatic advertising. In this post, we explore how MMM works, the benefits of MMM, and how it has evolved to become essential for advertisers making key, data-driven decisions in a privacy-conscious world.
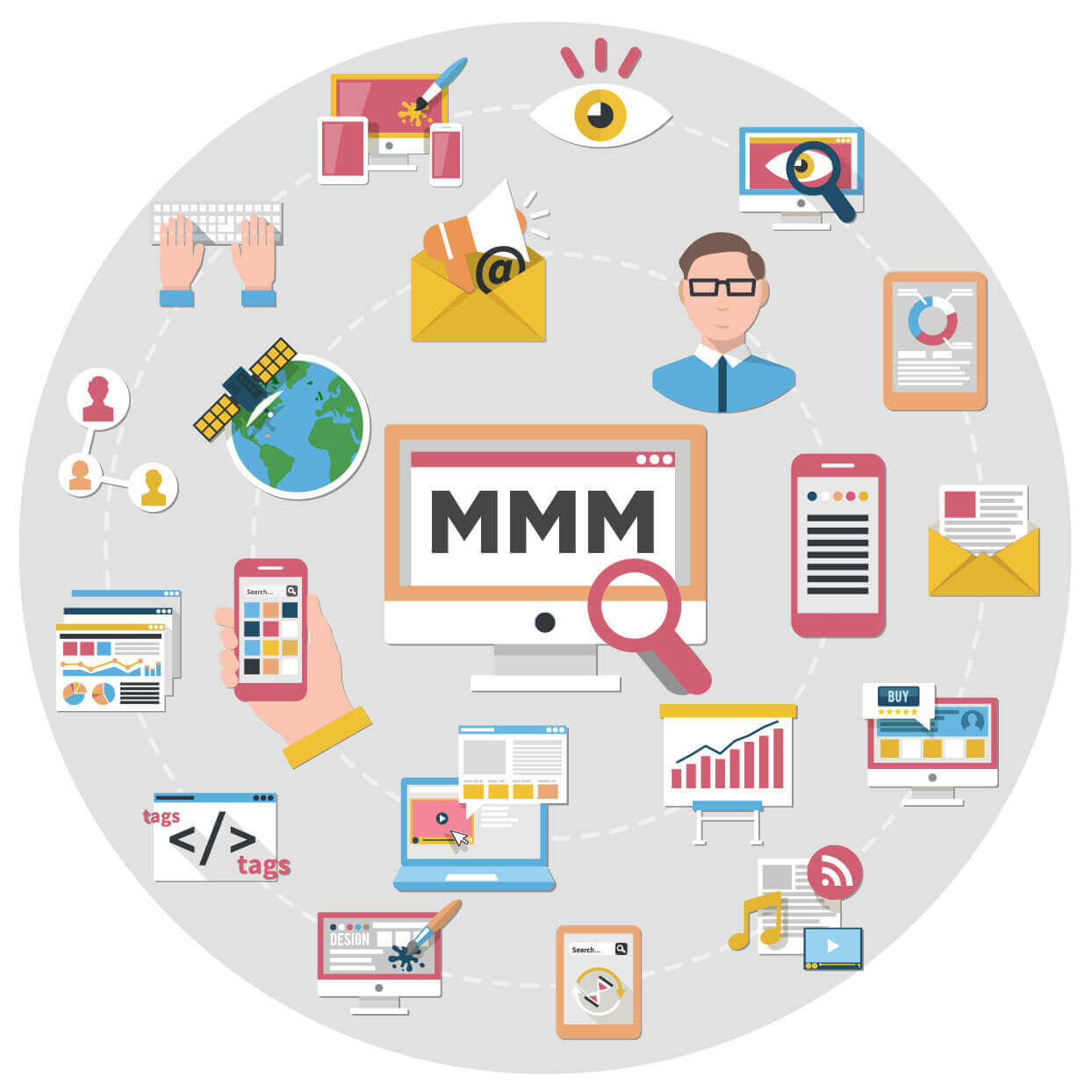
What Does MMM Stand For?
Known as marketing mix modeling or media mix modeling, MMM is a statistical data-analysis methodology that gives marketers a better understanding of the optimal mix of marketing strategies across media channels to positively impact sales and other key performance indicators (KPIs). MMM seeks to take into account all advertising channels—print, social, and online advertising (e.g., search, display, video) as well as offline channels.
How Does MMM Work?
Marketing mix modeling relies on aggregate data from marketing and non-marketing sources gathered over an extended period of time. Typically, a minimum of three or more months of historical data (ideally 12+ months) are necessary to reach data significance and account for seasonality shifts. This large volume of data is used to create an accurate demand model to give marketers insights into the most effective channel strategies and forecast the best omni-channel allocation of future ad spend for greatest impact and return on investment (ROI). From these insights, marketers can adjust ad spend allocation across channels and partners for future optimization.
Seems a little abstract? Let’s look at an example.
A CMO at a major fintech company wants to zoom out from granular campaign- and creative-level performance reporting to capture the bigger picture. The CMO’s goal is to understand the incrementality of ad spend across channels and overarching performance peaks and valleys throughout the year. They work with an MMM platform and after plugging in historical data are able to arrive at new recommendations for how much to spend across channel partners at different times of the year. The marketing director and UA manager now can reallocate spend across their various channel partners to drive better incrementality and reduce unwanted oversaturation on any given channel.
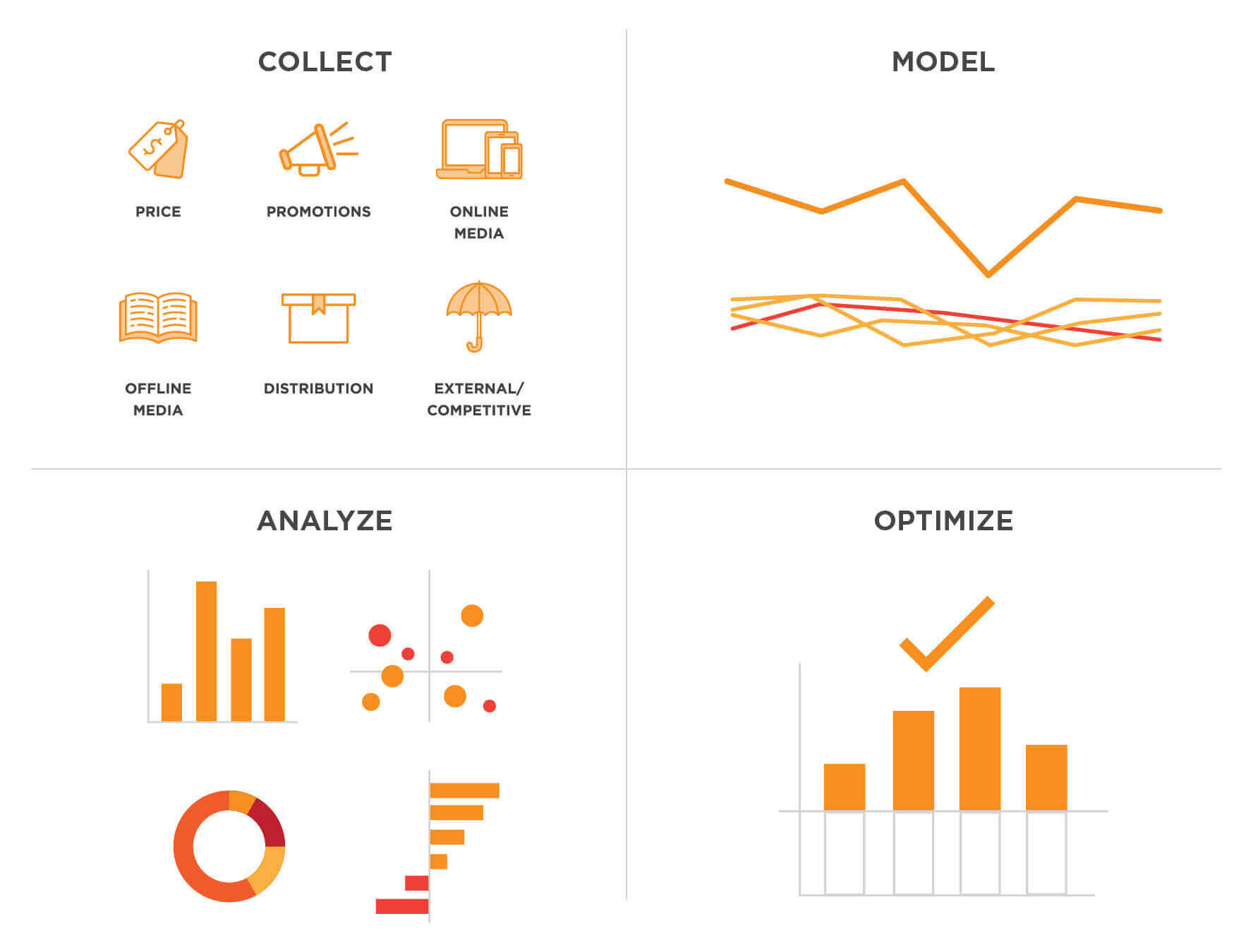
And that’s essentially MMM—collecting and processing a lot of data, then presenting it at a high, aggregated level so marketers can glean broad insights into advertising effectiveness, transcending individual outliers and skewed averages.
A Brief History of MMM
Marketing mix modeling is not new, but a marketing approach that has been utilized for decades. MMM took root in the 1950s and ’60s when marketers recognized the need for systematic approaches to measure and predict the relative impact of various marketing activities on sales. At the time, traditional media channels, including television, radio, and print advertising, dominated the landscape, and marketers customarily relied on basic tracking methods like surveys and sales data to evaluate and model their approaches. Iconic campaigns such as “Pepsi Generation” (1963), a persuasive lifestyle-brand initiative that targeted young adults, and McDonald’s “You Deserve a Break Today” (1971), which invoked convenience and an escape from routine, incorporated early MMM principles in their analysis of the interplay of elements such as advertising, pricing, and promotions and their relative impact on sales and customer behavior.
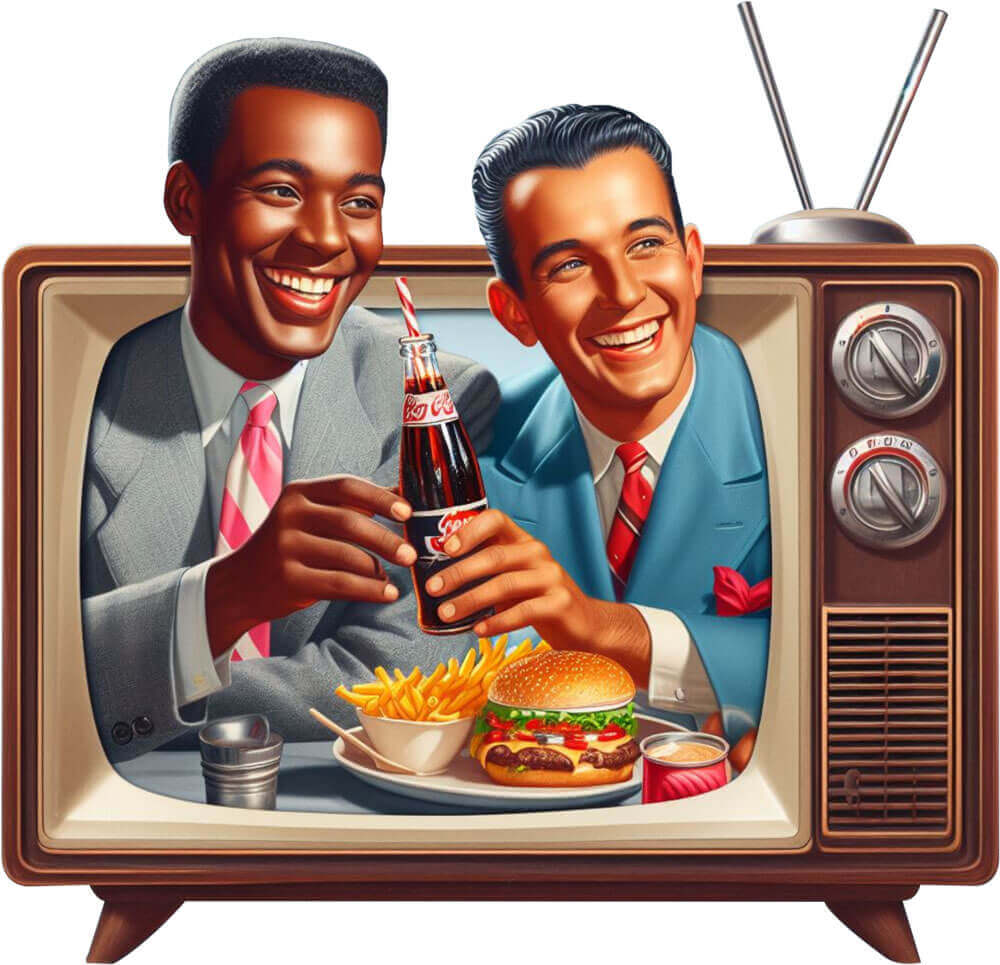
Early MMM pioneers faced challenging limitations in the computing power and data availability needed for this more complex marketing framework. As technological advancements in the 1980s enabled highly sophisticated methods of quantifying the effects of marketing variables, MMM came to full fruition. Over the next couple decades, MMM experienced a heyday. In particular, multinational consumer goods and food and beverage companies such as Nestlé, Procter & Gamble, and Coca-Cola, with their vast marketing resources, widely deployed intricate data-driven marketing analytics.
As digital marketing evolved in the early 2000s, MMM largely took a back seat to direct-response attribution modeling, which relies on user-level interactions on websites and mobile apps. Unlike MMM aggregated data, attribution data is inherently granular—useful for marketers in focusing their efforts on specific users and customers via direct response marketing. This approach facilitates insights derived from customer-level engagements, enabling marketers to drive creative optimization, A/B tests on messaging and creatives, and other personalized marketing tactics tailored toward unique persona profiles.
In recent years, however, MMM has seen a renaissance owing to the data processing and analysis potency enabled by AI and machine learning. Companies and their marketing teams have adopted the advanced analytics and predictive insights afforded by MMM to fuel growth. At the same time, recent developments in user privacy and data use have eroded the availability of granular, user-level attribution data. As a result, marketers are relying more on aggregated data and rediscovering the potential of MMM to inform their marketing strategy. MMM enables them to optimize budgets across channels while respecting privacy policies.
How MMM Is Evolving to Help Advertisers
With the revival of marketing mix modeling, how marketers interact with it has evolved to support the dynamic needs of today’s user acquisition teams. In the fast-paced digital advertising landscape, quarterly or semiannual MMM reports are quickly outdated and lack actionability. Traditional MMM is time-consuming and laborious to manage, making it accessible only to large organizations that have the resources to maintain it in-house or the budget to outsource it.
While historically only such corporations have been able to afford fully leveraging MMM, automated data flows, cloud computing, and machine learning have made MMM more accessible, accurate, nimble, and easily updated. Cutting-edge software as a service (SaaS) next-generation MMM solutions, now accessible to companies of all sizes, have been developed to fit the needs of today’s advertisers. AIM (Always-On Incremental Measurement) by Kochava, a real-time MMM tool, maximizes the effectiveness of the marketer’s budget by providing advanced control over incrementality, channel saturation, and seasonality. AIM utilizes a sophisticated learning system that ingests new data daily and continuously updates and enriches its models. This always-on approach ensures that the insights it produces never go stale and are always ready to use—providing marketers who must make confident decisions with turnkey recommendations for optimized budget allocations.
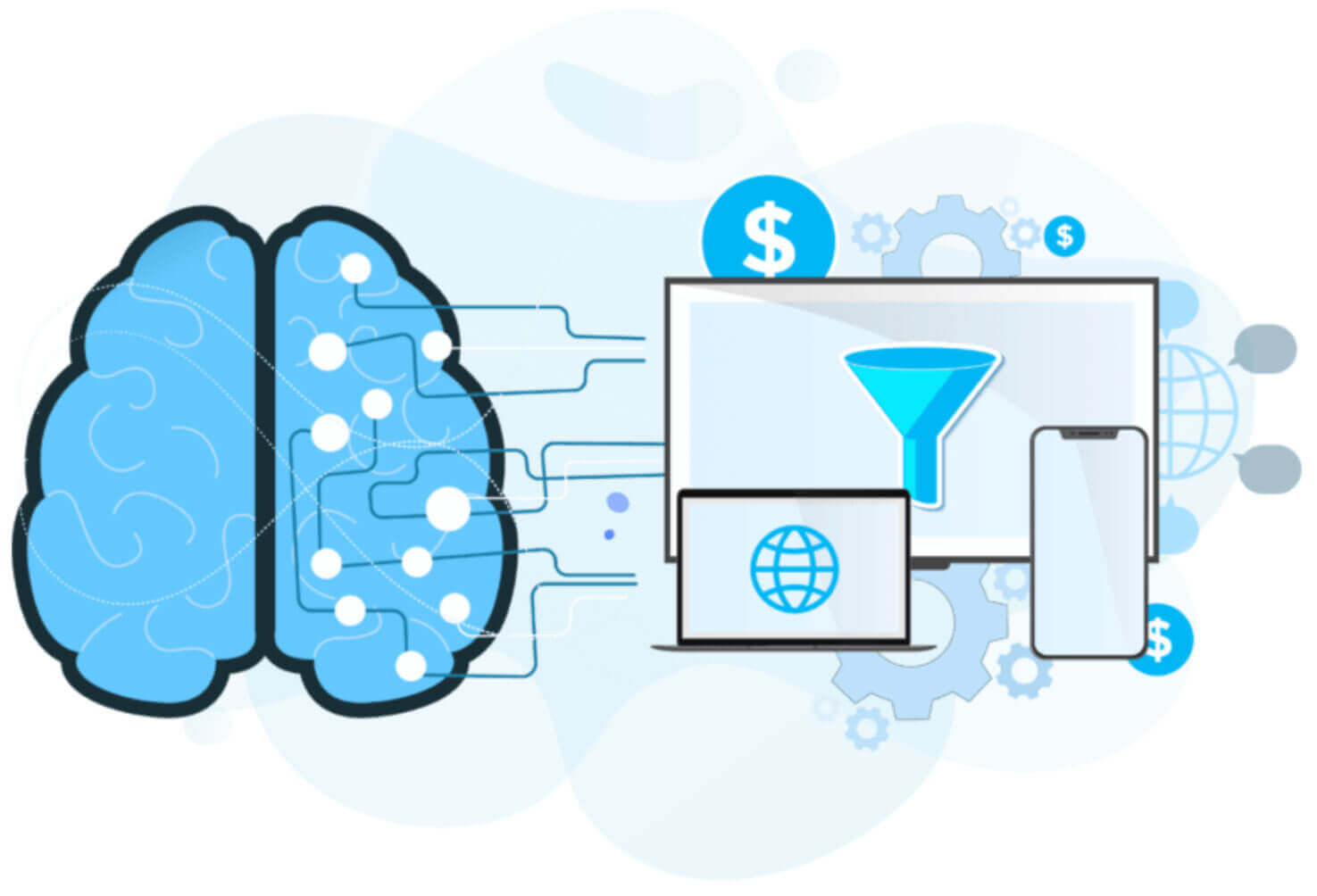
As user privacy continues to weave itself throughout the adtech ecosystem, next-generation MMM tools will become increasingly indispensable for advertisers in determining the effectiveness of their omni-channel media strategy.
The Conclusion on Marketing Mix Modeling
Next-generation MMM is at the forefront of a marketing revolution, offering actionable recommendations for data-driven decision making in an increasingly privacy-conscious adtech landscape.
Have questions or want more information on AIM and MMM? Check out our Marketing Mix Modeling 101 ebook and explore even more helpful content in the AIM Resource Center.
Subscribe to our newsletter to stay up to date on industry trends.